The Transportation Research Board (TRB) Annual Meeting in Washington, D.C. is one of the biggest annual events for transportation researchers, featuring thousands of transportation administrators, practitioners, policy makers, and researchers and more than 600 workshops, lectern sessions, committee sessions, and poster sessions.
Mobility Network was well represented at TRB's 102nd Annual Meeting January 8-12, 2023.
We are proud to showcase below some of the Mobility Network research and presenters at TRB 2023.
Guiding Principles for Planning Bus Transit Networks of Integrated On-demand and Scheduled Services
Alaa Itani (Supervisor: Prof. Amer Shalaby) (CivMin PhD 2024), Willem Klumpenhouwer, Brendon Hemily, Amer Shalaby
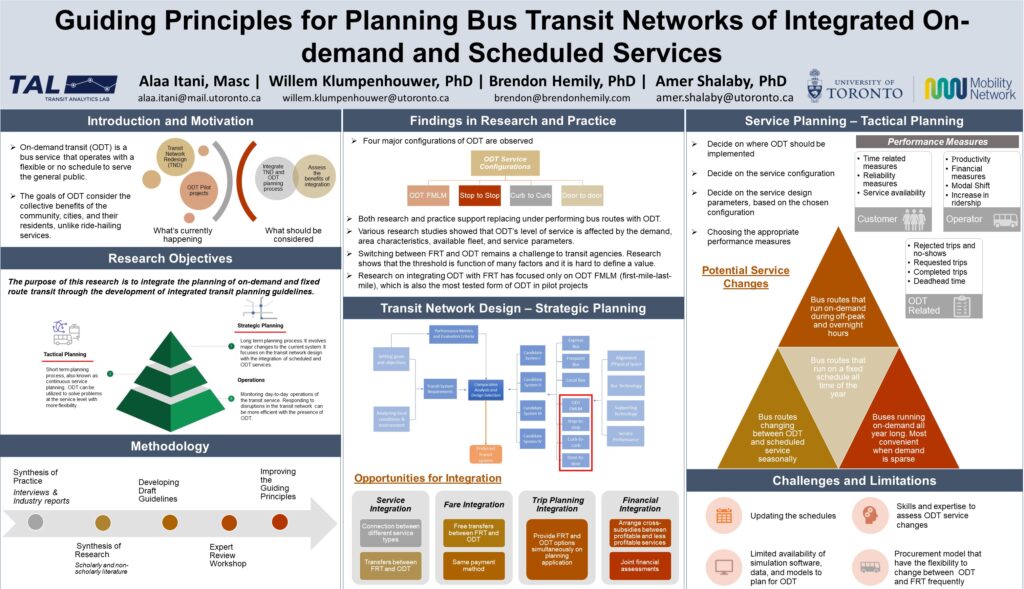
This research presents guidance on integrating on-demand transit (ODT) with conventional bus systems at the strategic and tactical planning processes. It is based on industry interviews, scholarly and non-scholarly research material, and an expert workshop hosting transit agencies in Canada that operate ODT. The results showed that there isn't a single recipe that works for all transit networks when it comes to integrating ODT with the fixed-bus systems.
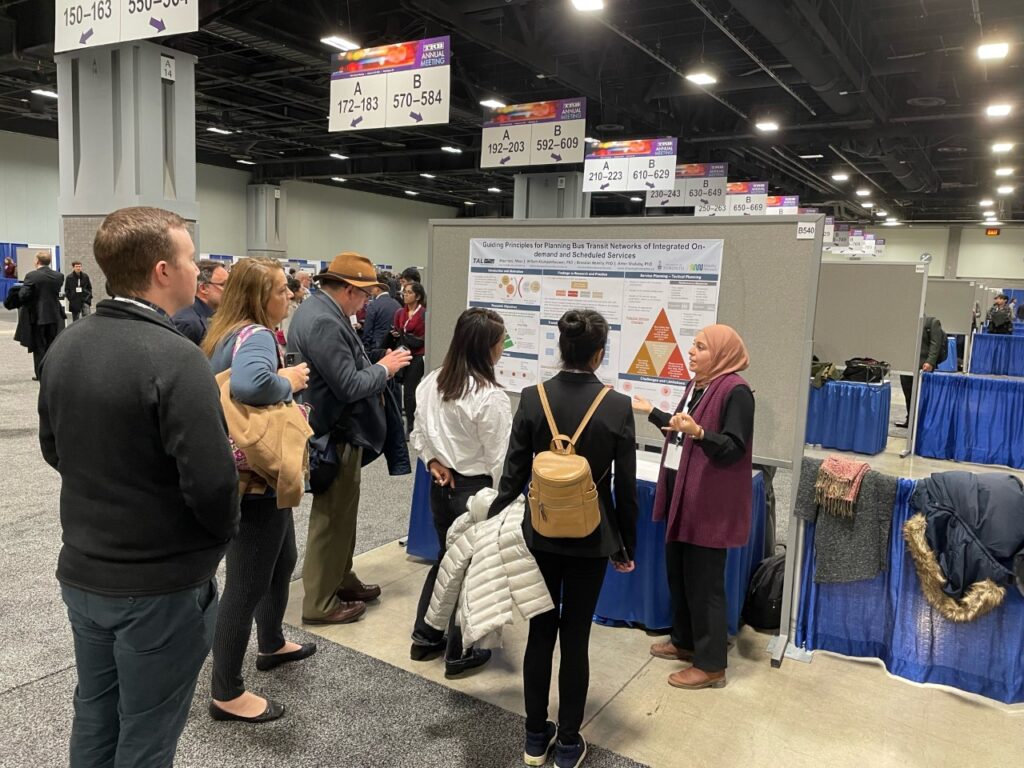
Exploring travel patterns of people with disabilities: A multilevel analysis of accessible taxi trips in Toronto, Canada
Yixue Zhang (Supervisor: Prof. Steven Farber) (Planning PhD 2023), Steven Farber, Mischa Young, Ignacio Tiznado-Aitken, Timothy Ross
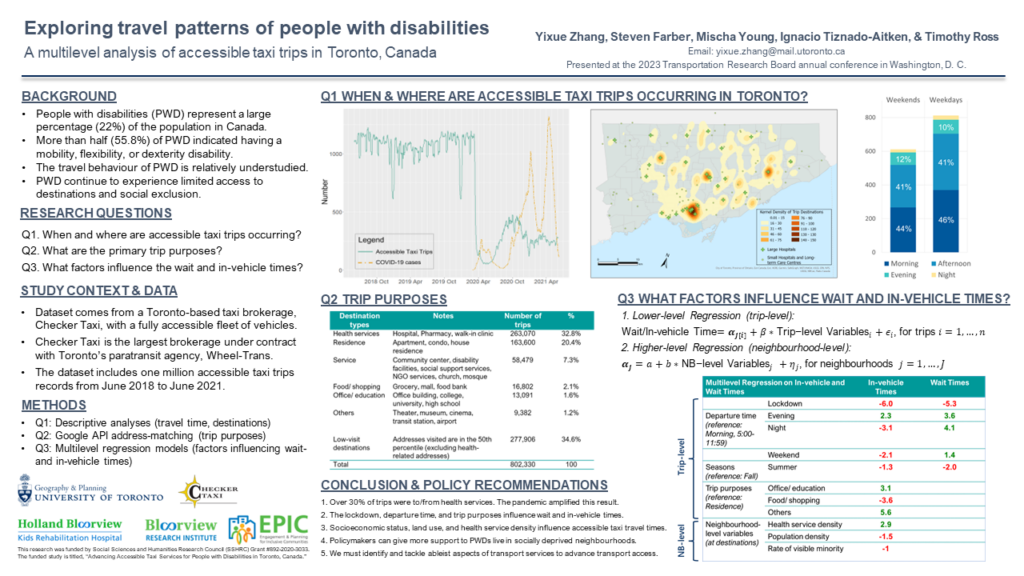
This study investigates how people with disabilities use accessible taxis in Toronto, Canada. Using spatial-temporal analyses and multilevel regression models, we found that about 33% of the accessible taxi users travelled to/from health-related services. Neighbourhood-level demographics also influence the in-vehicle travel times of accessible taxi trips.
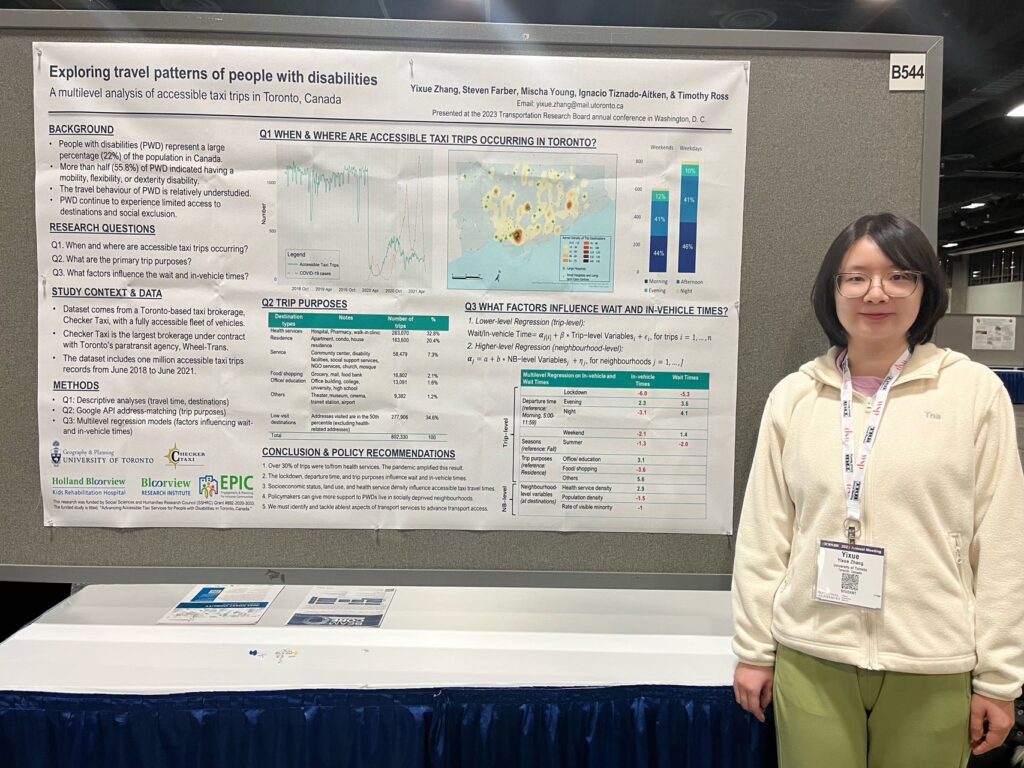
Novel Transit Management Strategies: Driver Advisory Systems with Cooperative Space and Time Priorities
Kareem Othman (Co-supervisors: Profs Amer Shalaby and Baher Abdulhai) (CivMin PhD 2024), Amer Shalaby, Baher Abdulhai
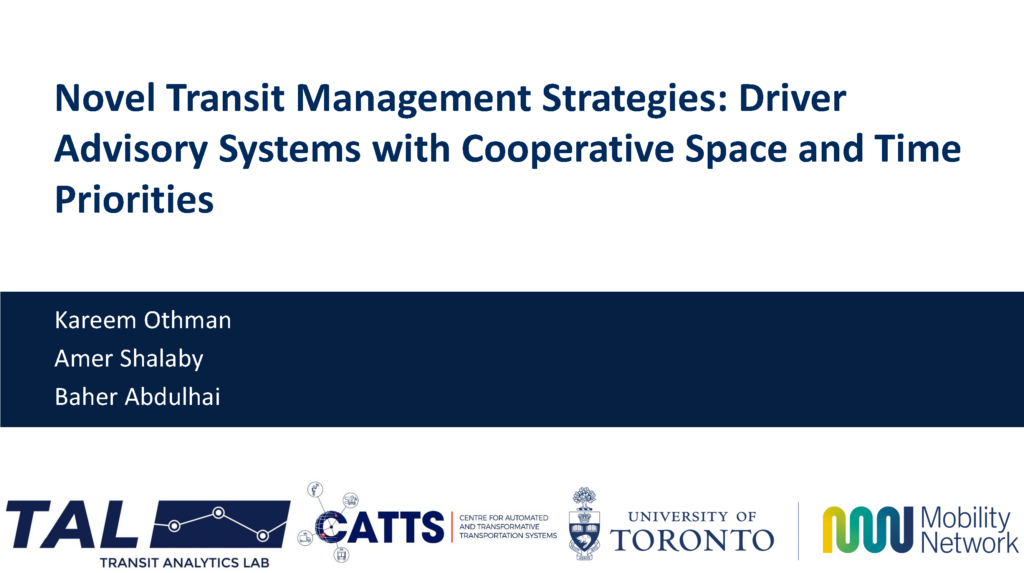
This study focuses on proposing novel transit management strategies that improve the entire corridor performance for both transit and traffic performance. The study focuses on analyzing the impacts of three main strategies on the corridor performance: Driver Advisory System, Space Priority (Exclusive Bus Lanes and Dynamic Bus Lanes), and Time Priority (Transit Signal Priority). The paper was presented in the "Best of Transit Management and Performance Papers" Lectern session in the TRBAM.
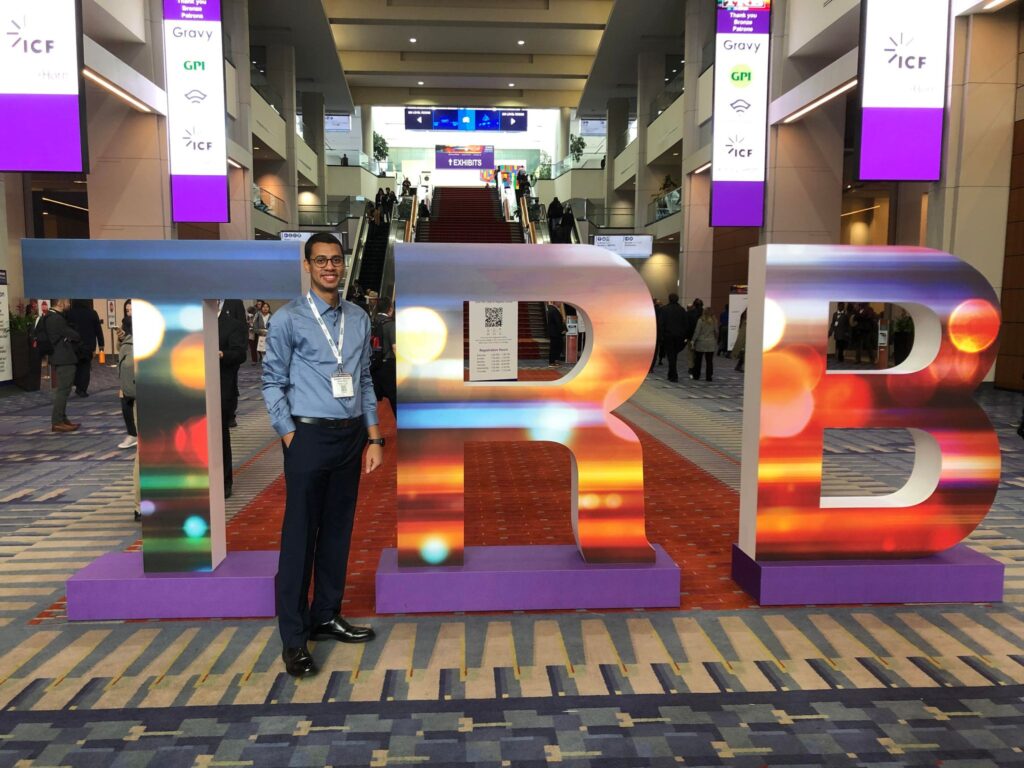
Constructing Origin-Destination Demand Matrix Using Wi-Fi and AFC Gate Count Data: A Case Study of Torontoโs Subway Network
Diego Da Silva (Supervisor: Prof. Amer Shalaby) (Postdoctoral Fellow, Transit Analytics Lab), Feras Elsaid, Amer Shalaby
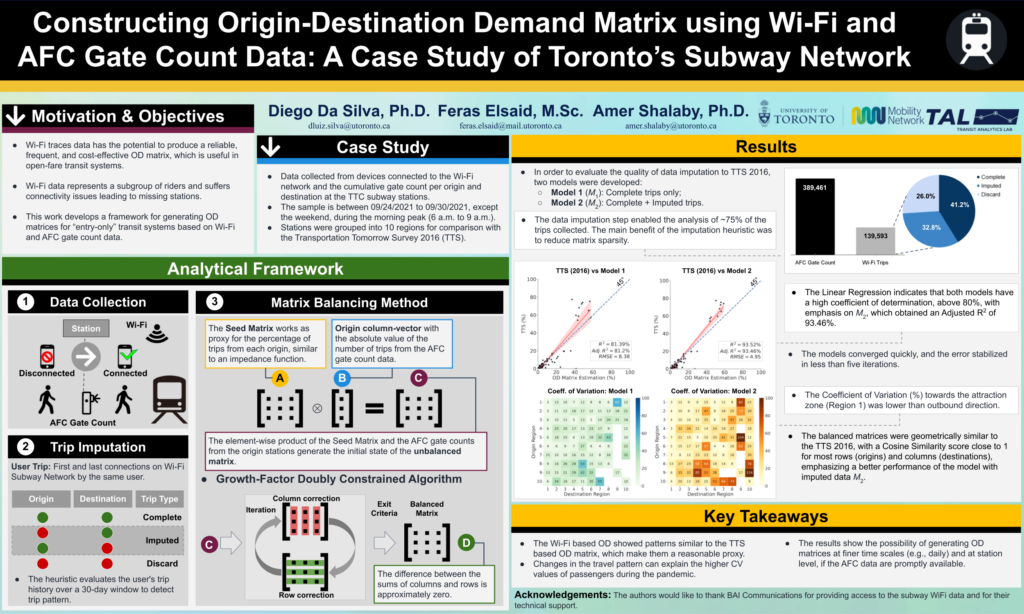
Our study is a framework for constructing OD matrices for entry-only urban rail transit (URT) systems using Wi-Fi traces and passenger counts at AFC entry/exit gates. It also proposed a heuristic for imputing missing journey information of incomplete Wi-Fi traces. The framework was demonstrated using the City of Torontoโs subway system as a case study.
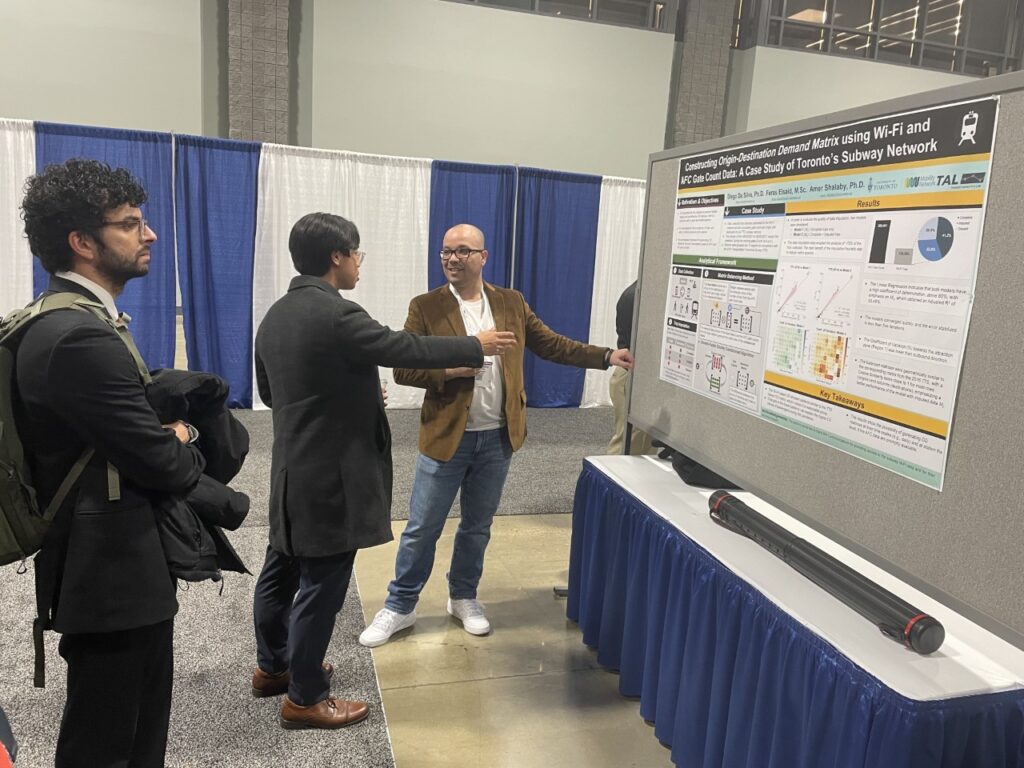
What can Bring Transit Ridership Back: An Econometric study on the Potential of Usage Incentives and Operational Policies in the Greater Toronto Area
Sk. Md. Mashrur, Kaili Wang, Brenden Lavoie (Supervisor: Prof. Khandker Nurul Habib) (CivMin MASc 2023), Khandker Nurul Habib
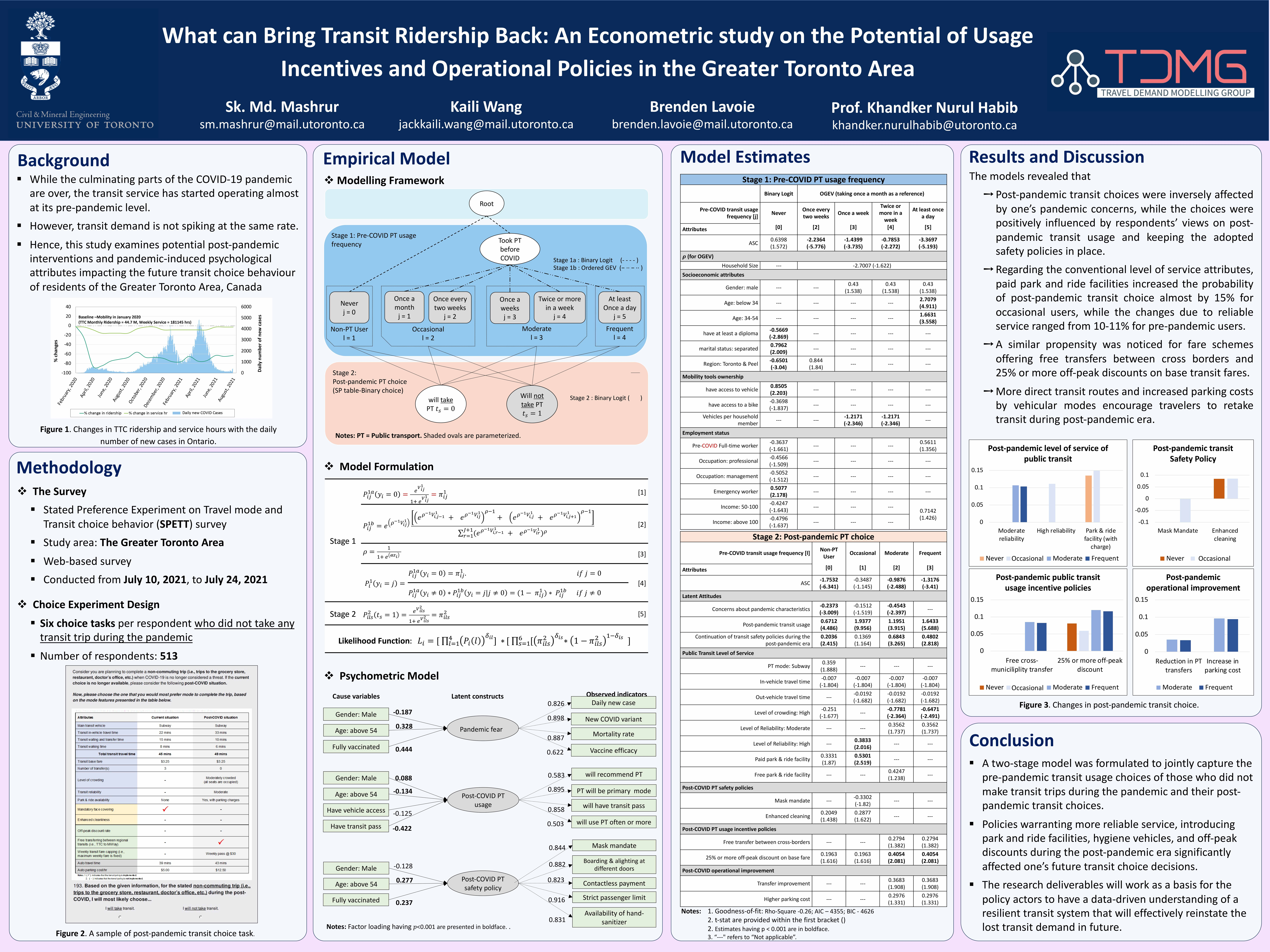
This research presents the findings of a transit demand and choice adaption survey of the GTA, with the goal of identifying potential actions transit agencies can take to bring riders back to the travel mode. A two-stage model was formulated to jointly capture the pre- pandemic transit usage choices of those who did not make transit trips during the pandemic and respective post-pandemic transit choices for these user groups.
Application of a Hybrid Choice Model to Assess the Influence of COVID-19 Risk Perceptions on Transit Route Choice in the Greater Toronto Area
Sk Md Mashrur, Kaili Wang, Khandker Nurul Habib
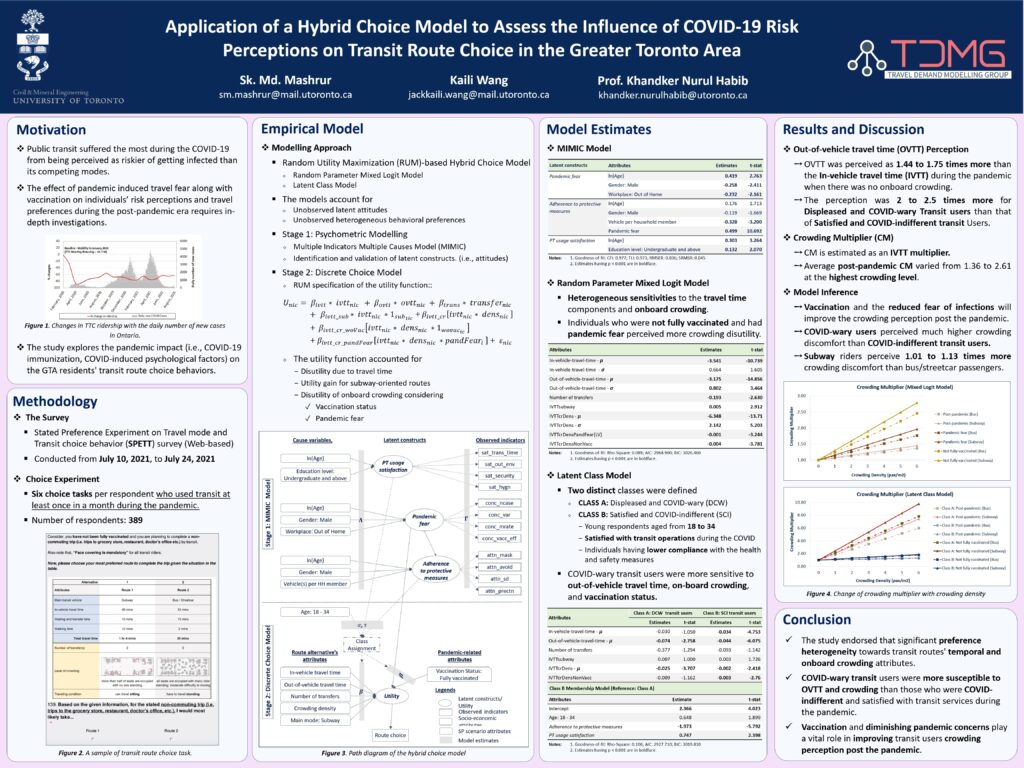
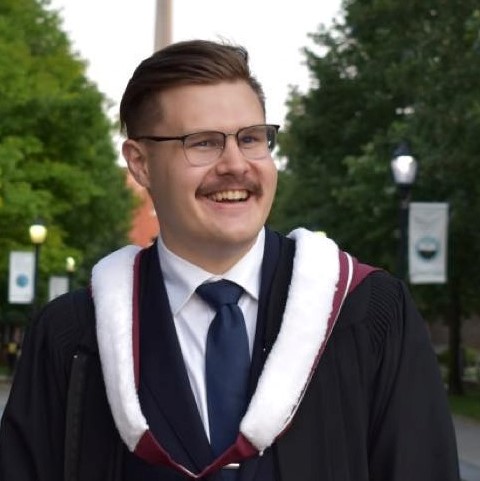
eMARLIN: Distributed Coordinated Adaptive Traffic Signal Control with Topology-Embedding Propagation
Xiaoyu Wang (Co-supervisors Profs Baher Abdulhai and Scott Sanner) (CivMin PhD 2024), Ayal Taitler, Ilia Smirnov, Scott Sanner, Baher Abdulhai
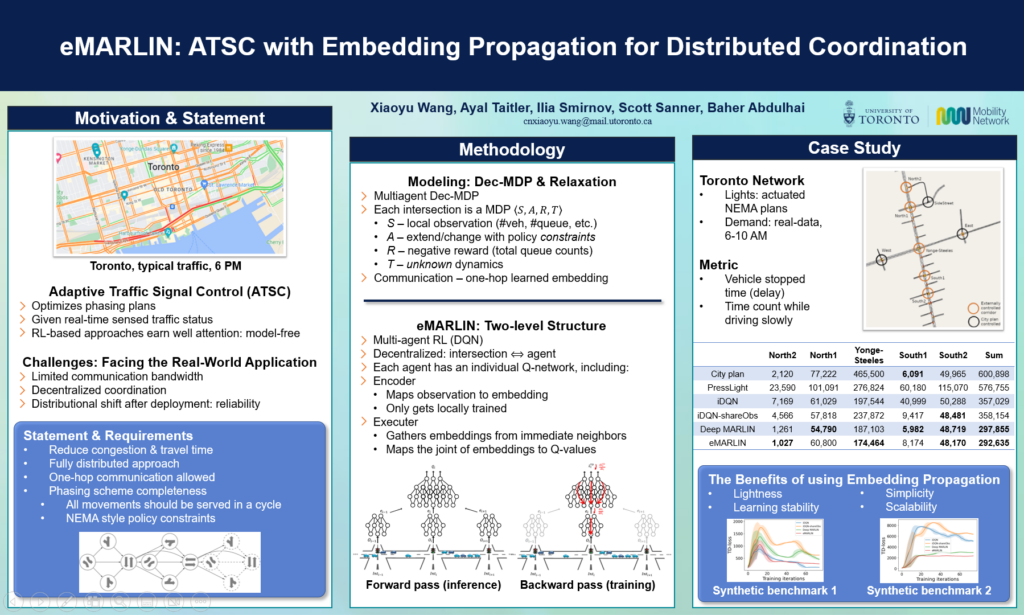
In this work, we study the problem of adaptive traffic signal control and coordination to increase the efficiency and throughput of surface traffic networks. We seek a fully decentralized approach to accommodate the potential infrastructure limitations in the field. eMARLIN, a deep reinforcement learning method, is designed to achieve intersection-wise coordination while the communication bandwidth is restricted.

Methods for Analyzing Subway System Performance and User Experience Using WiFi Connection Data
Aidan Grenville (Supervisor: Prof. Amer Shalaby) (EngSci Aerospace BASc 2023), Willem Klumpenhouwer, Natalie Chui, Amer Shalaby
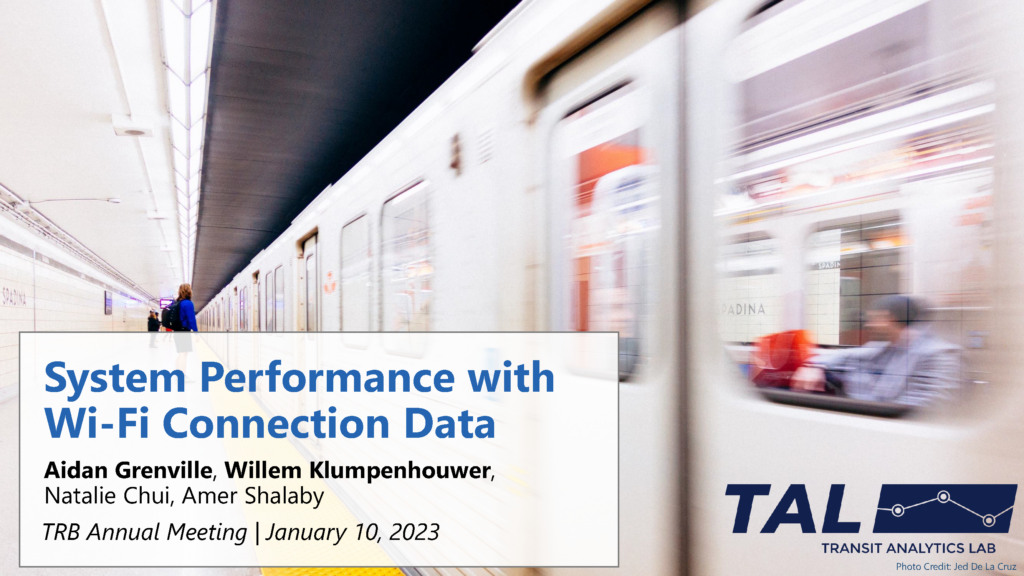
Typical performance measurements of public transit operations make use of vehicle-based data such as automated vehicle location data, or passenger-based data at specific fare collection points. Ideally, the performance of a transit system from a reliability and passenger experience should be measured through individual passenger journeys. The growing prevalence of smartphones provides one potential source for this analysis, as passive methods such as WiFi, cellular, and Bluetooth connection data allow us to observe devices as they move throughout the system, measuring various aspects of customer experience and reliability, including methods for detecting train arrivals at platforms, estimating wait times, and analyzing origin-destination travel time variation.
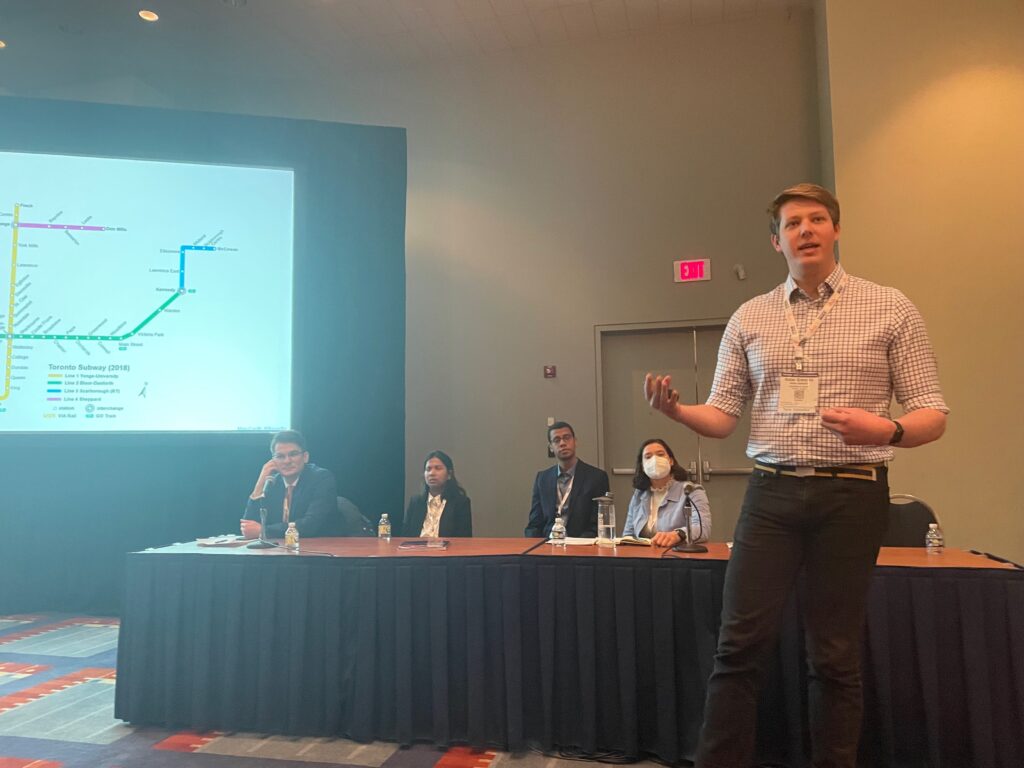
Rational Inattention in Discrete Choice Models: Estimable Specifications with Empirical Applications of RI-Multinomial Logit (RI-MNL) and RI-Nested Logit (RI-NL) Models
Khandker Nurul Habib, PhD, P.Eng.
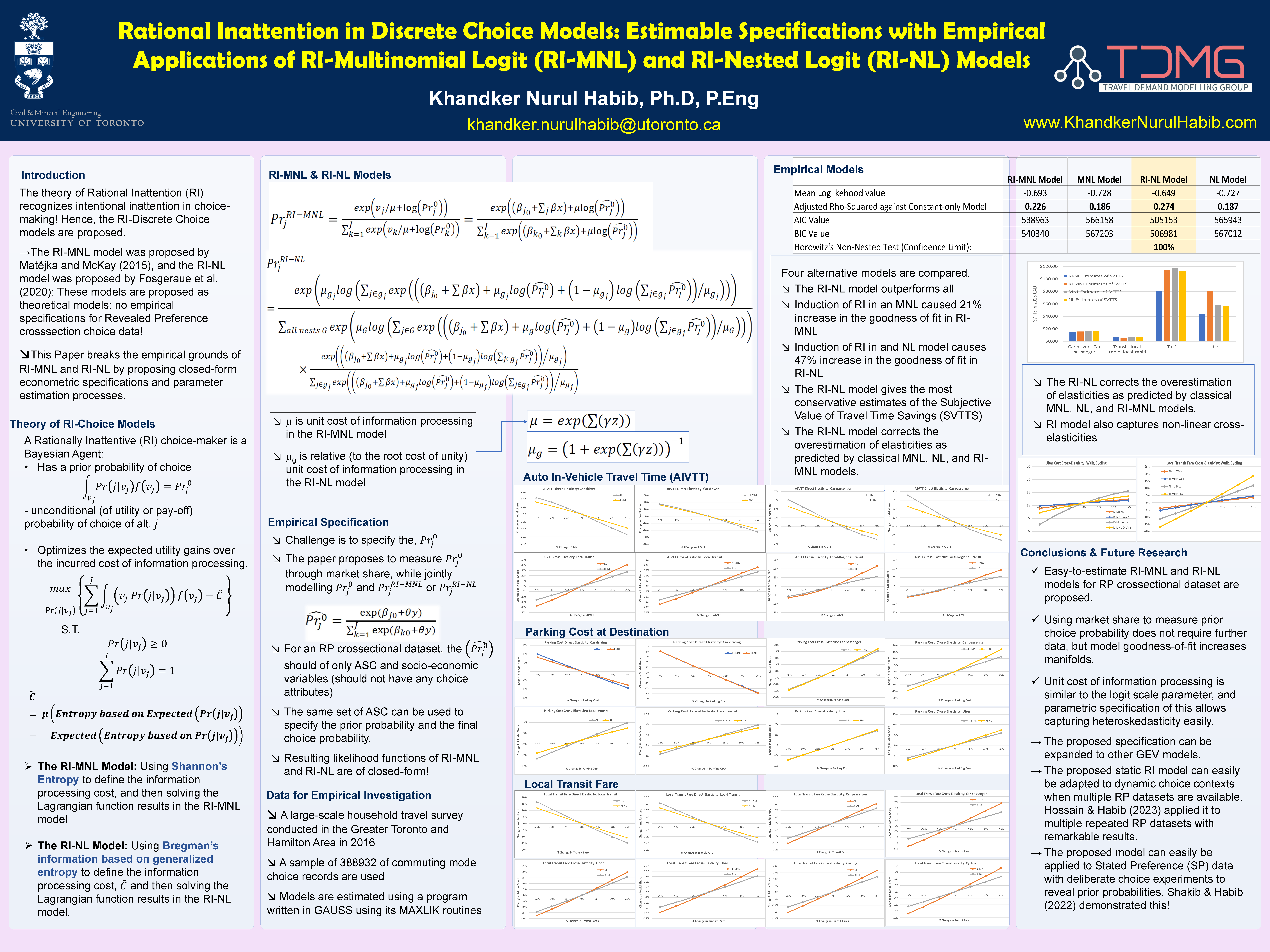
As opposed to the fully informed choice-making assumption in classical discrete choice models, the theory of Rational Inattention (RI) in discrete choice modelling has been recently proposed in the literature. Matฤjka and McKay (2015) proposed the RI-multinomial logit (RI-MNL), and Fosgerau et al. (2020) proposed the RI-nested logit (RI-NL) model. These models consider that choice makers are bayesian agents with prior probabilities of choices and process any further information at the cost of an information processing cost to have the updated/posterior choice probabilities. However, the proposed RI-MNL and RI-NL models are theoretical formulations without any estimable empirical specifications. This paper proposes econometric formulations of RI-MNL and RI-NL models that are estimable using classical maximum estimation methods and suitable for revealed crossectional choice data. The proposed models are estimated for commuting mode choices in the Greater Toronto and Hamilton Area (GTHA) using data from a household travel survey conducted in the region. Empirical investigation reveals that the induction of RI in the classical discrete choice models (MNL and NL) improved the model fit by large margins. While just scale parameterization in classical MNL and NL does not make a better model, the scale parameterization better captures the choice heterogeneity within the RI framework. Between the RI-MNL and RI-NL, the RI-NL is proven to be the best. The RI-NL model can capture asymmetric (between increasing and decreasing values) elasticities of choice attributes.
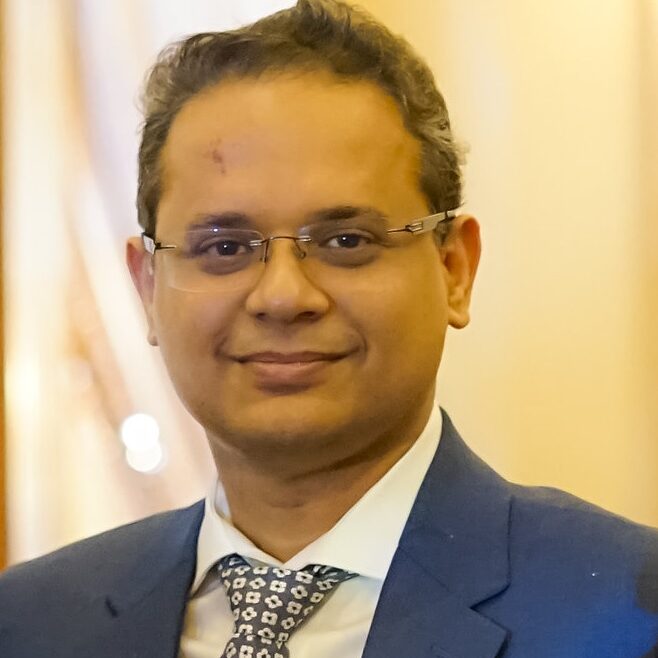
An Electric Cargo Tricycle Pilot Study
Usman Ahmed (Supervisor: Prof. Matthew Roorda) (CivMin Postdoctoral Fellow), Matthew Roorda
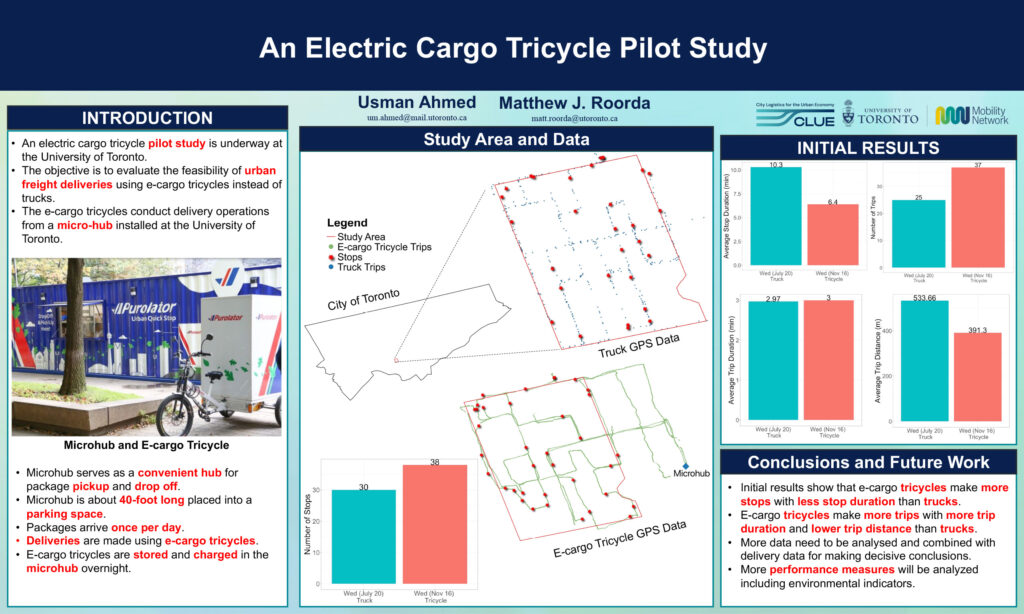
A pilot study is underway at the University of Toronto where urban freight delivery operations using e-cargo tricycles are compared with trucks via a before/after study. Preliminary analysis of the pilot were presented at TRB 2023.
Firm Location Choice Model Considering Firm Membership
Usman Ahmed (Supervisor: Prof. Matthew Roorda) (CivMin Postdoctoral Fellow), Jason Hawkins, Matthew Roorda
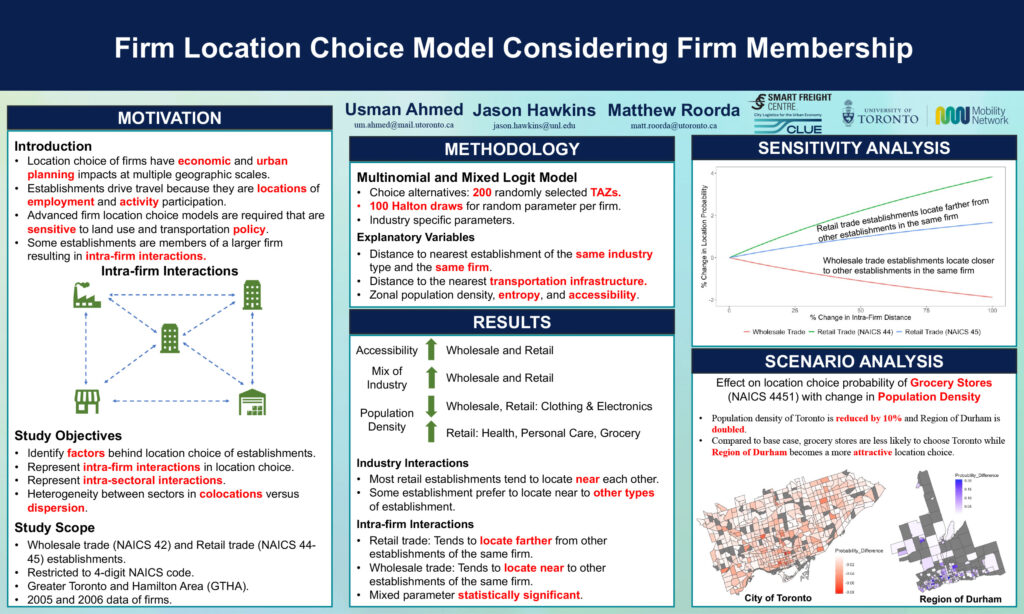
Location choice of firms have economic and urban planning impacts at multiple geographic scales. A model of firm location choice considering intra-firm interactions was presented at the TRB.
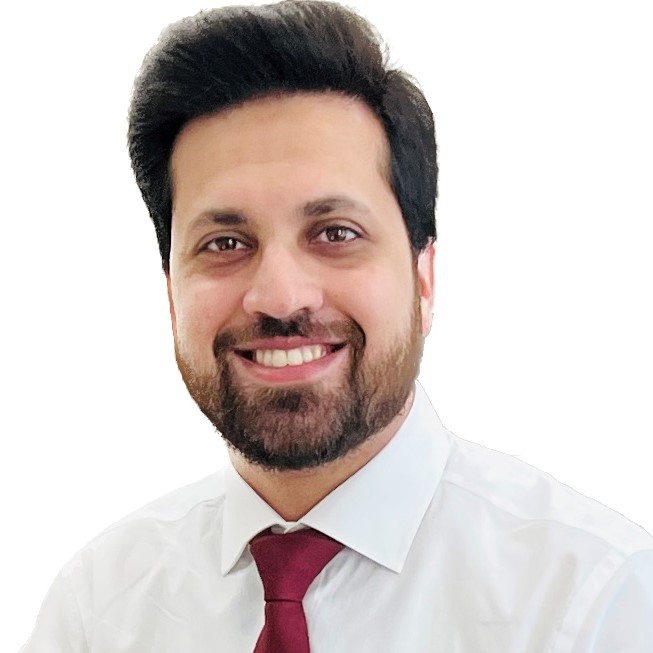
Fusing Repeated Cross-sectional Revealed Preference Datasets based on Rational Inattention Theory: Accounting for Changing Modal Preferences
Sanjana Hossain (CivMin PhD 2022) (Supervisor: Prof. Khandker Nurul Habib), Khandker Nurul Habib
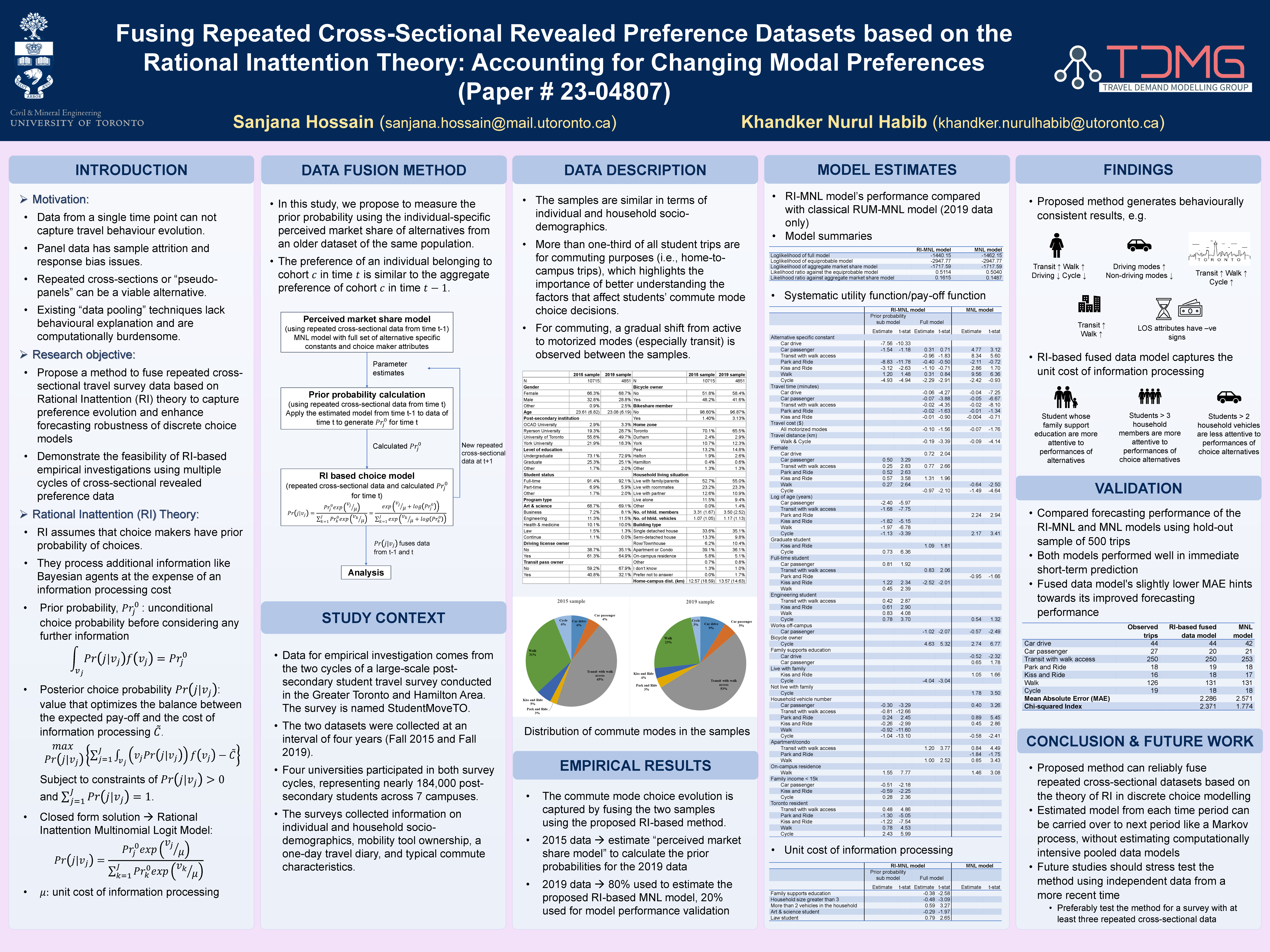
To address the methodological limitation of cross-sectional studies and the data constraints of longitudinal/panel studies, this research presents a model-based method to fuse repeated cross-sectional travel survey data based on the theory of Rational Inattention in discrete choice modelling. In the proposed framework, older cross-sectional data is used to model the prior probability of choice alternatives, and more recent cross-sectional data is used to capture conditional heterogenous choices. The fusion method is theoretically more robust and computationally less burdensome than existing data pooling techniques.
A Comprehensive Data Fusion to Evaluate the Impacts of COVID-19 on Passenger Travel Demands: Application of a Core-Satellite Data Collection Paradigm
Sanjana Hossain (CivMin PhD 2022) (Supervisor: Prof. Khandker Nurul Habib), Patrick Loa, Kaili Wang, Sk. Md. Mashrur, Alireza Dianat, Khandker Nurul Habib
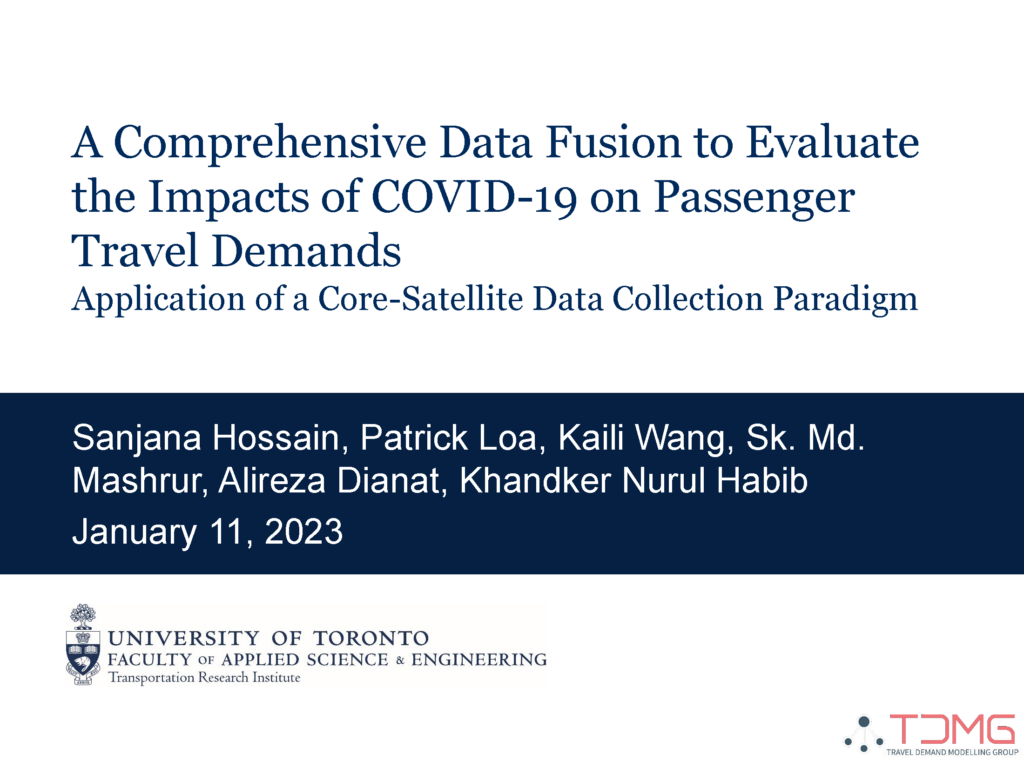
This research demonstrates the feasibility of fusing a โcoreโ household travel survey with three specialized โsatelliteโ surveys to evaluate the impacts of COVID-19 on passenger travel demand in the Greater Toronto Area. The study uses a non-parametric implicit data fusion method to generate multiple synthetic datasets that contain observed travel diaries and socioeconomic attributes of the trip-makers from the core survey, along with imputed attitudinal statements based on the satellite surveys. The results highlight the ability of the method to sufficiently reproduce the distribution of the attitudinal variables and the ability of the imputed variables to support the estimation of an advanced econometric model.
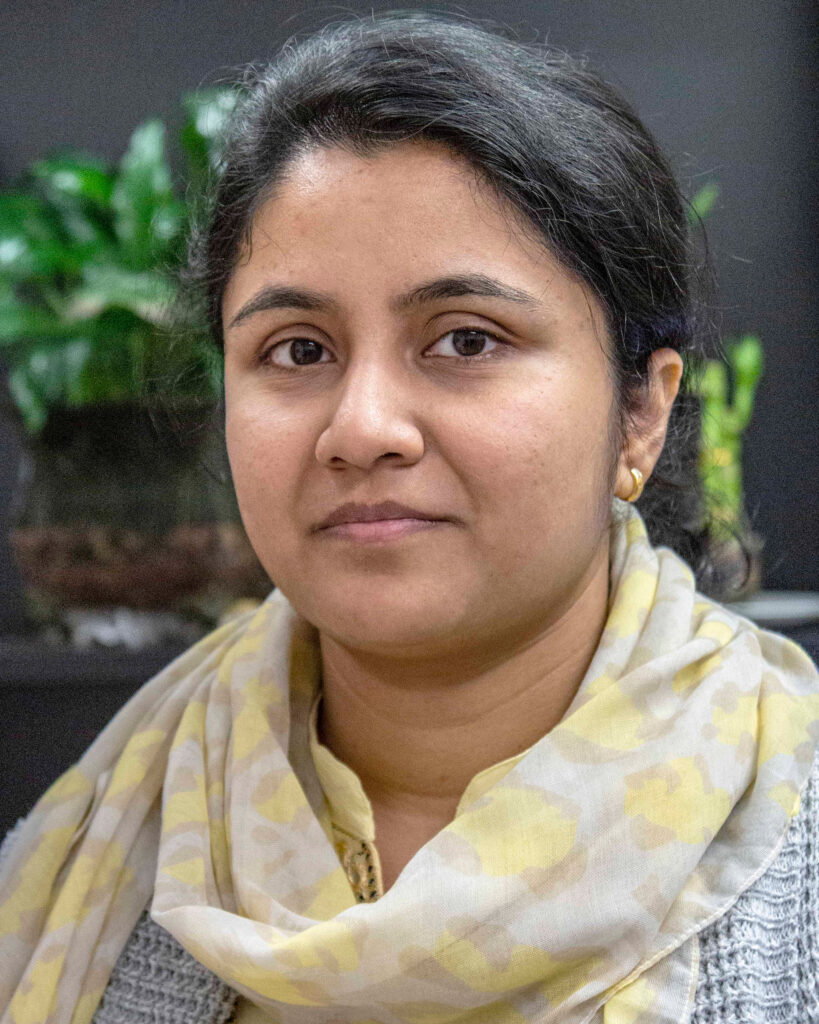
Identifying Profiles of Ride-sourcing Users in the Metro Vancouver Region for a Better Understanding of Ride-sourcing Behaviour
Patrick Loa (Supervisor: Prof. Khandker Nurul Habib) (CivMin PhD 2023), Felita Ong, Khandker Nurul Habib
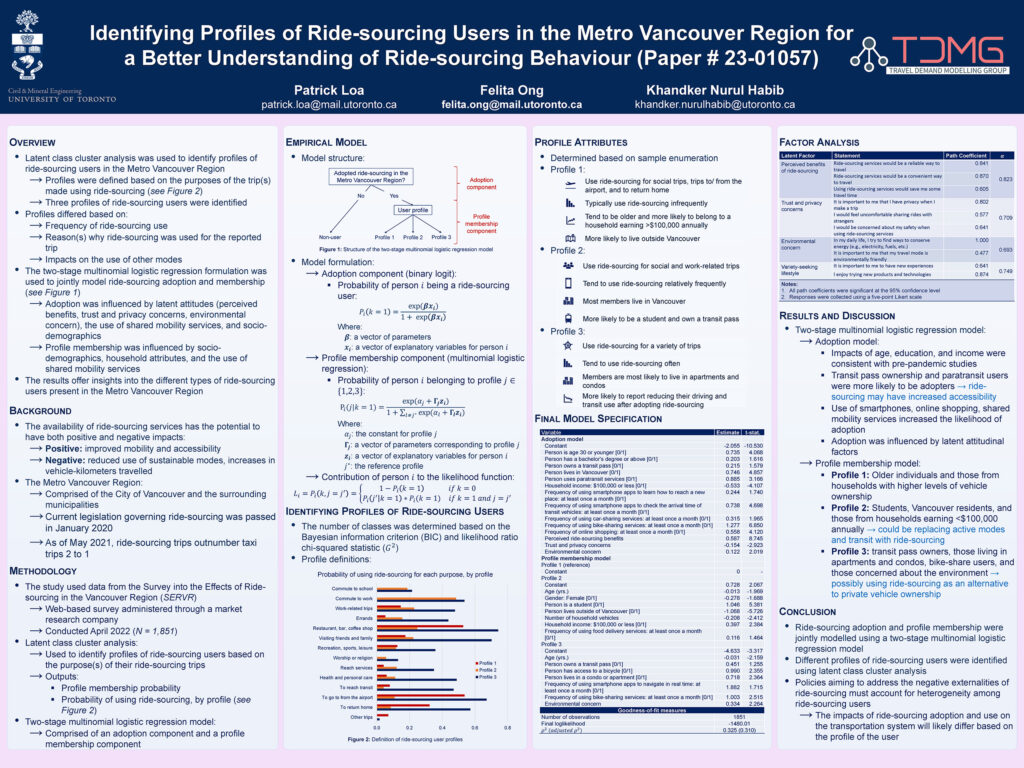
Using data from a web-based survey of residents of the Metro Vancouver Region, we used latent class cluster analysis to identify profiles of ride-sourcing users based on the purpose(s) for which they use these services. As part of our analysis, we developed a joint model of ride-sourcing adoption and profile membership. The results highlight the influence of socio-demographic attributes, mobility tool ownership, and attitudinal factors on the adoption and use of ride-sourcing.
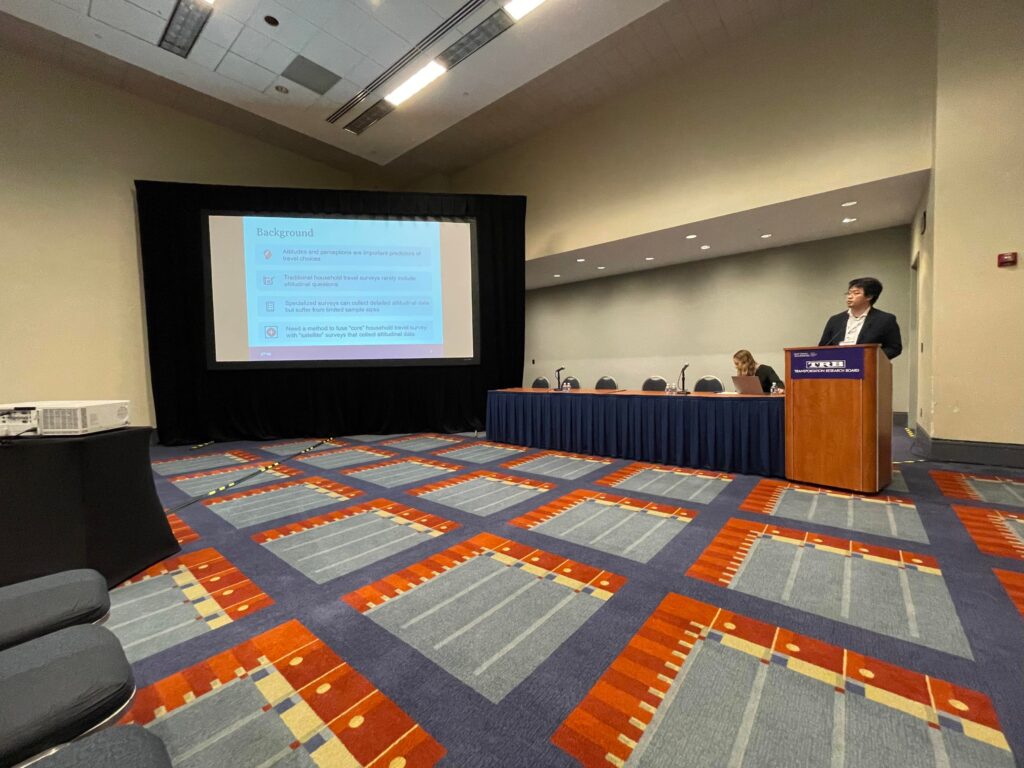
Improving truck driver and vulnerable road user interactions through driver training: An interview study with Canadian subject matter experts
Alia Galal (Co-supervisors Profs Matthew Roorda and Birsen Donmez) (CivMin PhD 2024), Birsen Donmez, Matthew Roorda
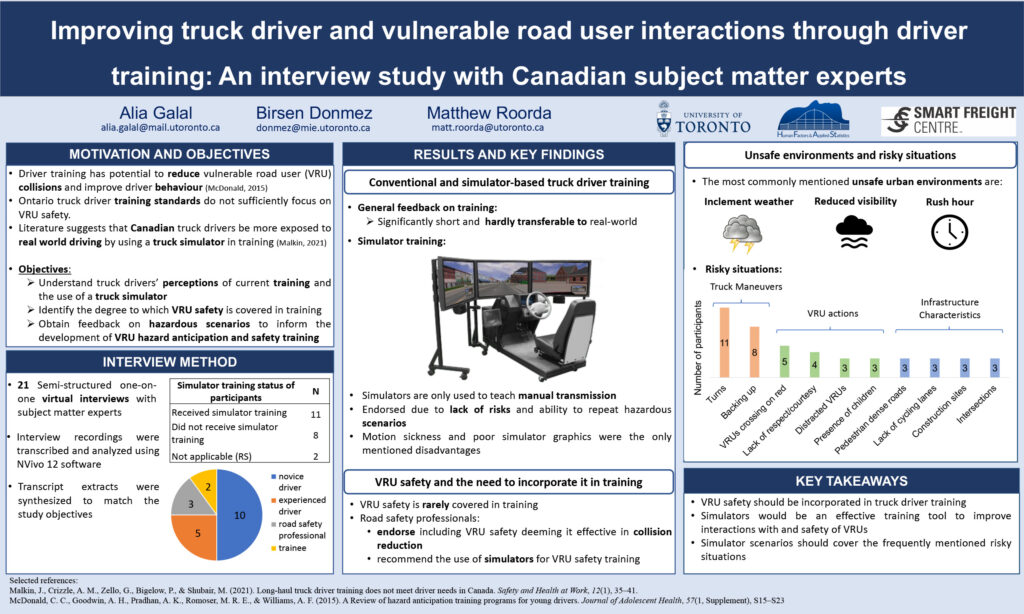
In this interview study, the aim is to understand subject matter expertsโ perceptions of current truck driver training in Ontario to identify gaps and potential improvements, particularly targeting vulnerable road user safety in urban areas. We also investigate the degree to which VRU safety is covered and the potential to incorporate it in training using a simulator.
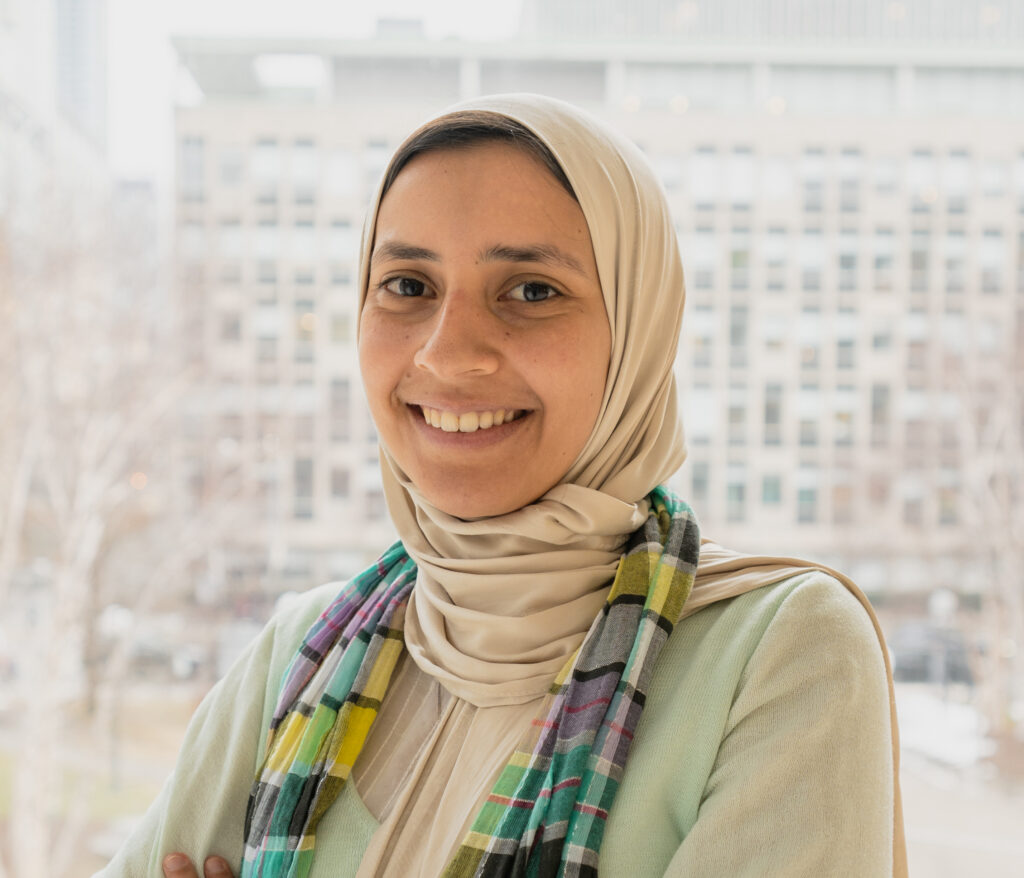
Internet and Communication Technology (ICT) Expansion and Continuation During the COVID-19 Pandemic: Evidence from The Greater Toronto Area
Alireza Dianat (Supervisor: Prof. Khandker Nurul Habib) (CivMin PhD 2024), Kaili Wang, Khandker Nurul Habibโ
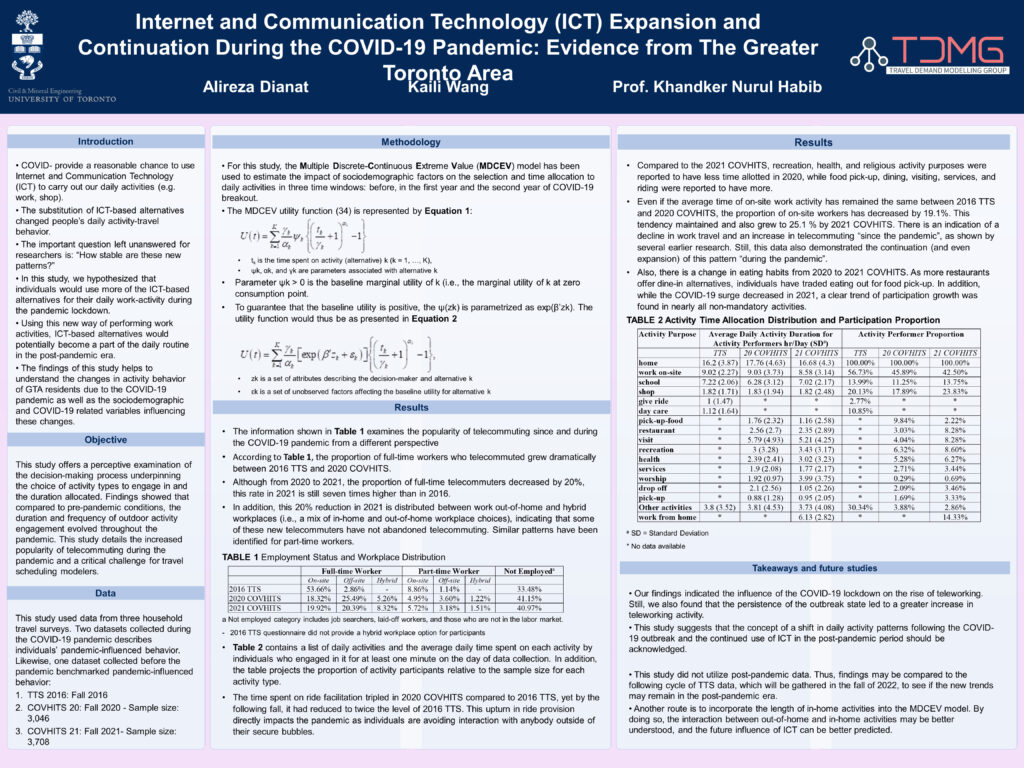
In this study, we hypothesized that individuals would use more of the ICT-based alternatives for their daily work-activity during the pandemic lockdown.โ Using this new way of performing work activities, ICT-based alternatives would potentially become a part of the daily routine in the post-pandemic era.โ The findings of this study helps to understand the changes in activity behavior of GTA residents due to the COVID-19 pandemic as well as the sociodemographic and COVID-19 related variables influencing these changes.
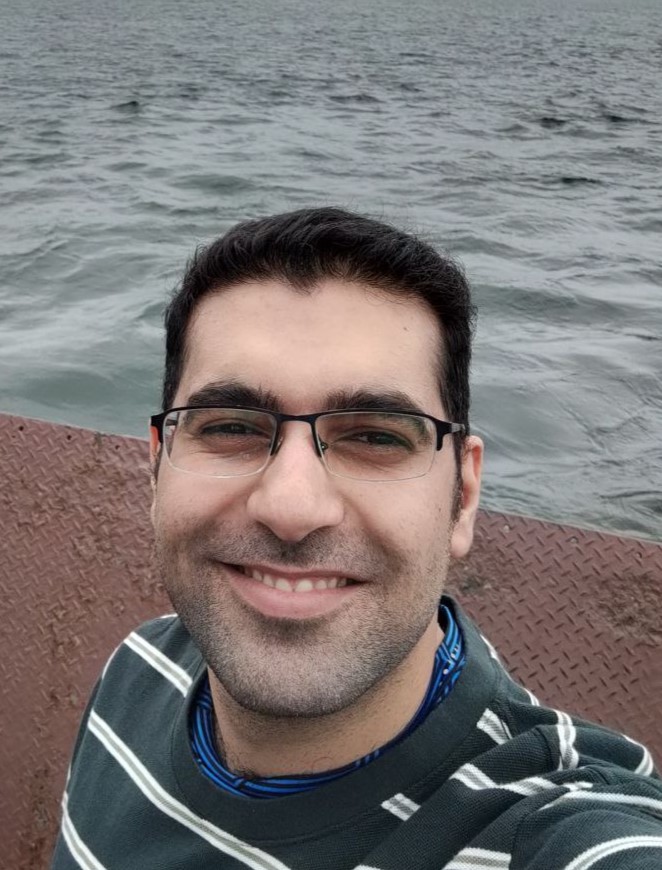
Using Twitter to Gauge Customer Satisfaction Response to a Major Transit Service Change in Calgary, Canada
Rami Al-Sahar, Willem Klumpenhouwer (Supervisor: Prof. Amer Shalaby) (Postdoctoral Fellow, Transit Analytics Lab), Amer Shalaby, Tamer El-Diraby
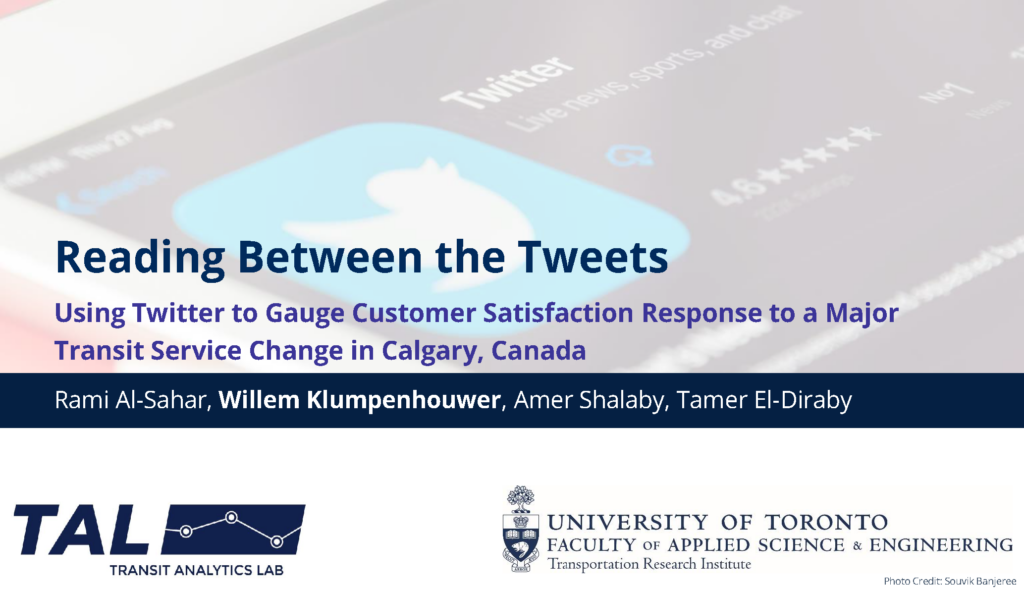
We compared both the on-time performance and transit-focused Twitter sentiment of public transit before and after a major service change in Calgary, Canada. We found that for adjusted routes, performance decrease corresponded with a decrease in the sentiment about specific routes, and a connection exists between low-performing sections of a route and the negative sentiment expressed online.
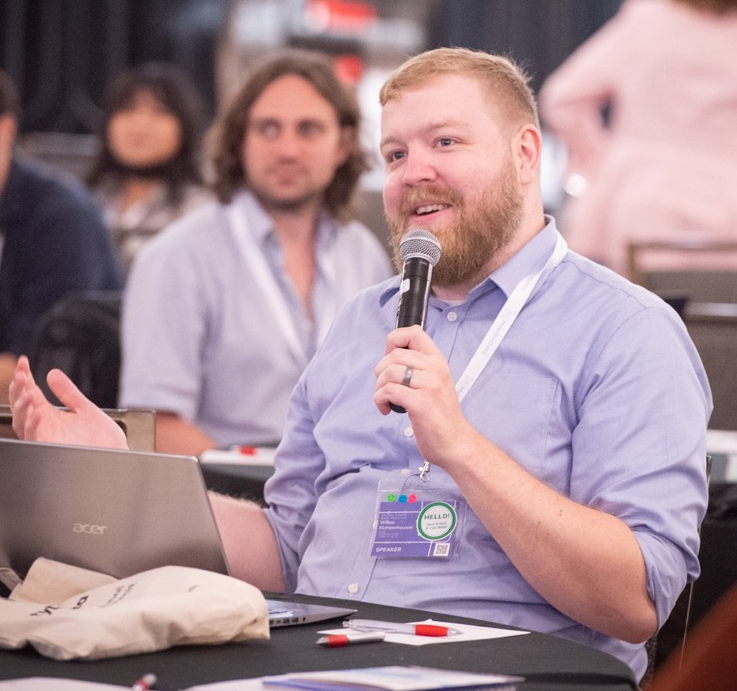
A Multitask Learning Deep Neural Networks Model of Stated Preference Analysis: exploratory analysis on changes in Ride-Sourcing utilization during the Covid-19 pandemic in Toronto
Yicong Liu (Co-supervisors: Profs Eric Miller and Khandker Nurul Habib) (CivMin PhD 2025), Patrick Loa, Kaili Wang, Khandker Nurul Habib
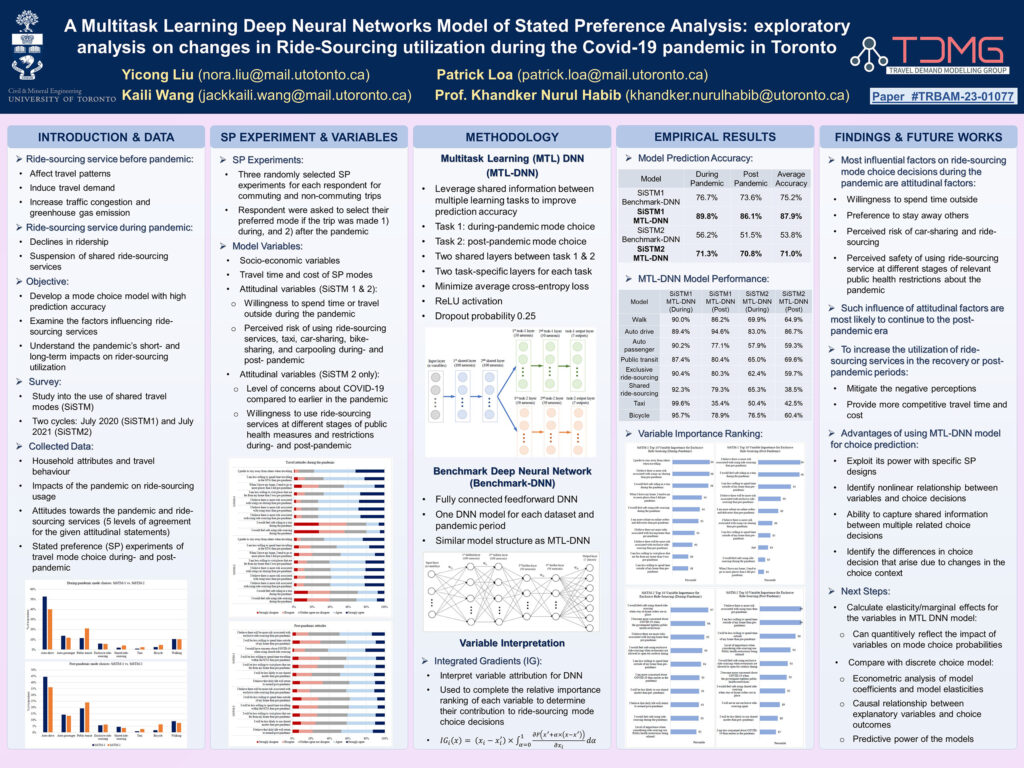
In this study, we developed a mode choice model using a novel structure of deep neural network. The model achieved high prediction accuracies, and was used to examine factors influencing people's mode choice decision, particularly the use of ride-sourcing services. It can also be utilized to understand the pandemicโs short- and long-term impacts on rider-sourcing utilization. We discovered that people's perceived safety of using ride-sourcing services and willingness to spend time outside are determining factors.
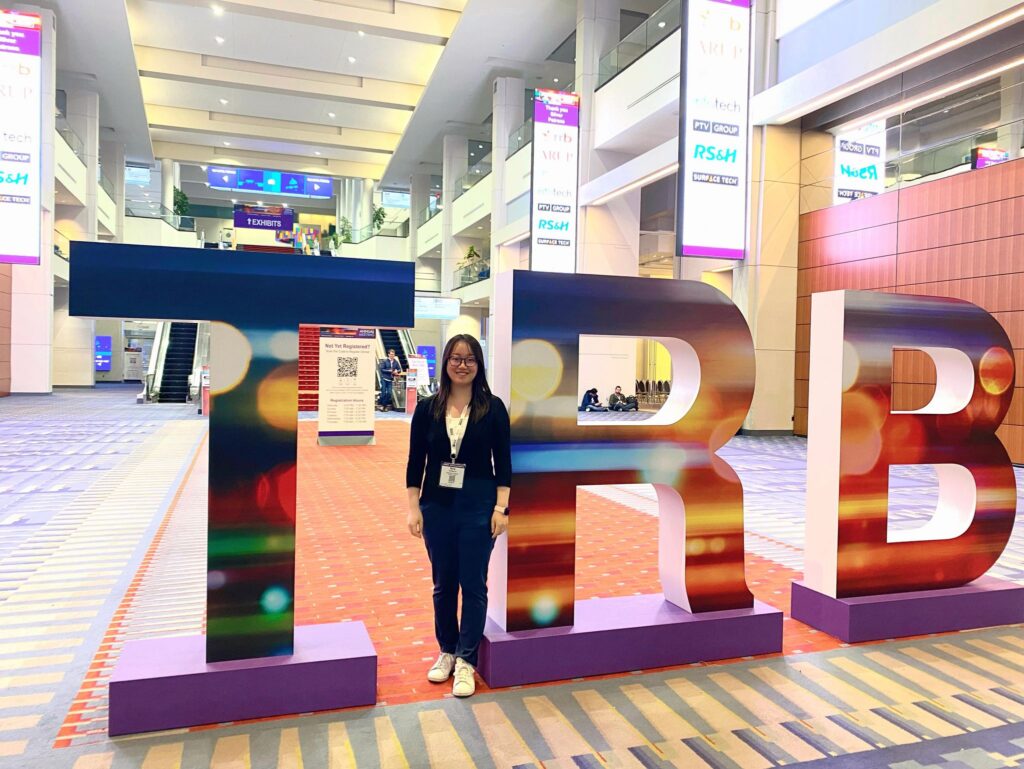
Impact of Transit Delays on Equity Seeking Groups in Toronto: A Time Expanded Graph Approach
Rick Liu, Amer Shalaby
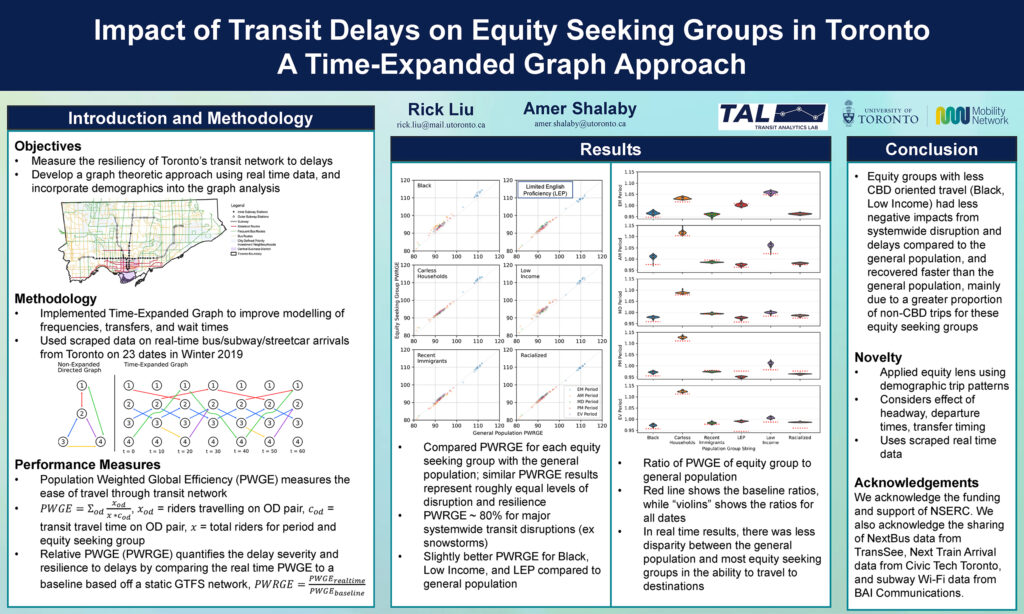
This paper reports on the analysis conducted by Rick Liu for his MASc thesis, under the supervision of Professor Amer Shalaby. The study employed graph theory techniques to evaluate the efficiency of Torontoโs transit network. Using global efficiency, which measures the ease with which riders can reach their destinations, we explored if equity seeking riders suffer the same level of travel time delays as the general population during both minor (recurrent) service disturbances and major (non-recurrent) disruptions.
Investigation of the interaction between urban rail ridership and network topology characteristics using temporal lagged and reciprocal effects: A case study of Chengdu, China
Mengwei Xin, Amer Shalaby
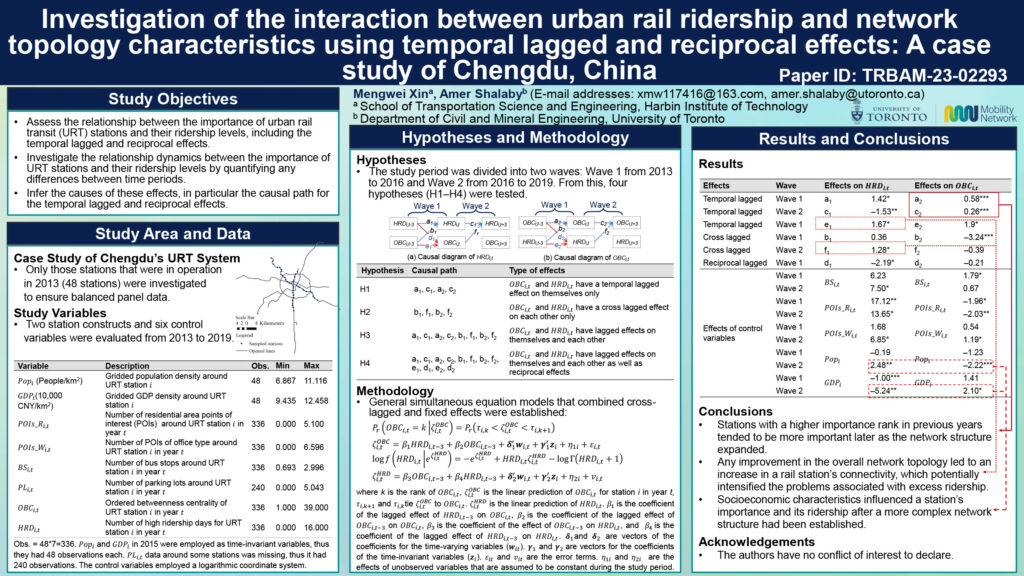
This paper reports on the analysis conducted by Mengwei (Vivian) Xin as a visiting PhD student at the University of Toronto under the supervision of Professor Amer Shalaby. The study assessed the relationship between the importance of urban rail transit (URT) stations and their ridership levels, including the temporal lagged and reciprocal effects. The study investigated the dynamics in the relationship between the importance of URT stations and their ridership levels.
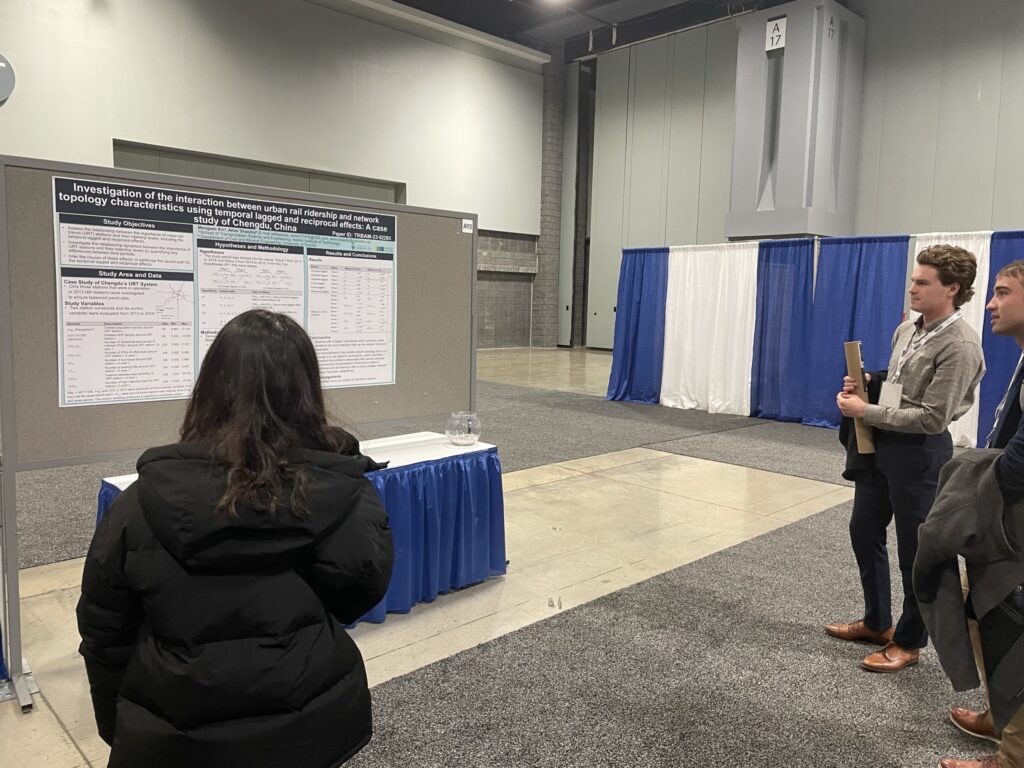
Panel Regression Analysis of Disruption Frequency of Urban Rail Transit Systems
Jinyi Chen, Amer Shalaby, Tiezhu Li, Hui Liu, Yiyong Bo, Fei Lin
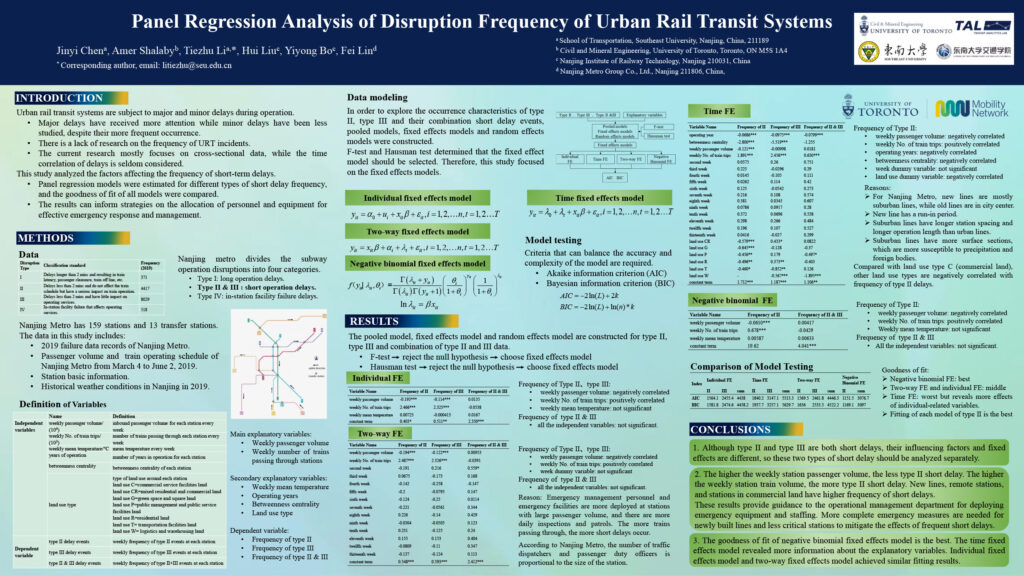
This paper reports on the analysis conducted by Jinyi Chen as a visiting PhD student at the University of Toronto under the supervision of Professor Amer Shalaby. This study analyzed the factors affecting the frequency of short-term delays. Panel regression models were estimated for different types of short delay frequency. The results can inform strategies on the allocation of personnel and equipment for effective emergency response and management.
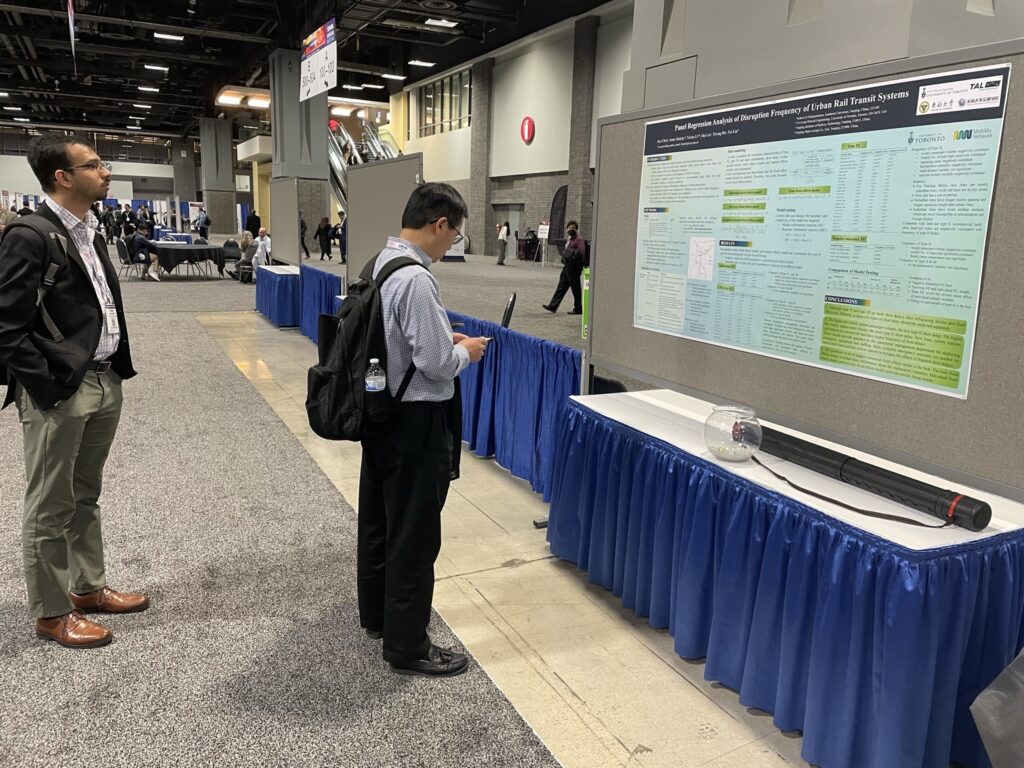
Dr. Amer Shalaby presented the above three posters at TRB 2023.